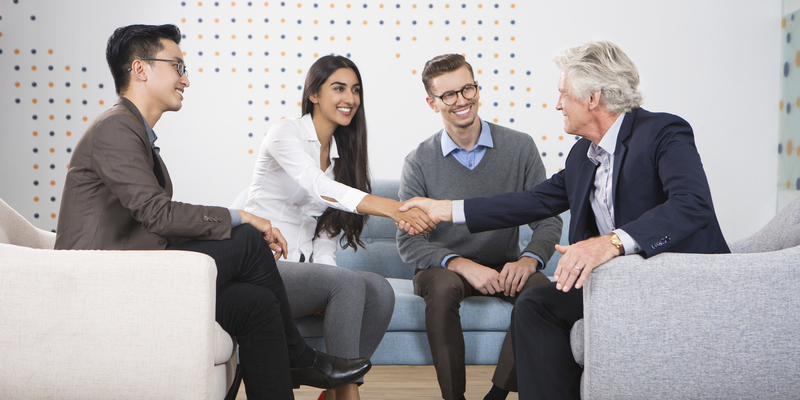
Artificial intelligence (AI) in HR isn't new. It's been around for years, helping leaders optimise their workdays in various ways. What is new, however, is generative AI.
As generative AI makes its way into HR departments, leaders should be equipped to have meaningful and accurate discussions about it. Understanding how generative AI differs from common types of AI in HR, particularly extractive AI, is a good start.
Unlike generative AI, extractive AI has existed for a long time and may already be at work in your organisation. When discussing AI, you may need to specify whether you're referring to extractive or generative capabilities, especially if you plan to discuss them with AI experts.
What is extractive AI?
Extractive AI pulls relevant information from a source, such as a document or database, and presents it in a readable format.
"Extractive AI can help you find a paragraph or certain words and phrases in a source," says Fernando Schwartz, vice president, data science and machine learning engineering, ADP. "It directly extracts the information and delivers it to you; however, it's not necessarily delivered in a human context."
Organisations have used extractive AI for a while to create forecasts and manage or analyse HR data, such as job applications in applicant tracking systems (ATSs), employee performance reviews and sentiments from employee surveys.
What is generative AI, and how is it different?
Generative AI is a recent development that uses large language models (LLMs) to generate human-like responses to user queries, or prompts. A popular example is the generative AI chatbot. Unlike extractive AI, generative AI can create new content from a source, such as a text- or image-based dataset. Generative AI tools are trained on vast data pools, can understand the context of a user's prompt and create images and text responses that mimic human conversation.
"Generative AI tools understand text and instructions in context and can communicate them more humanly," Schwartz says. "They not only extract information but convert it into images and natural-sounding responses, making you feel like you're conversing with an informed coworker."
Leaders should prioritise generative AI in HR. It is the newer of the AI technologies and has the most untapped workforce potential. It can optimise HR task completion, educate people — a win for learning and development professionals — answer HR questions and much more.
Choosing HR solutions with generative AI features
When choosing a generative AI HR solution, it's crucial to consider the experience and data of the vendor. Organisations that have been working with AI for longer and that have more extensive datasets are likely to have robust generative AI solutions capable of handling a broader range of use cases. Compliance, data privacy and data security are also important considerations, especially when sensitive information such as employee data is involved. Leaders should consider where their HR vendor stores this data, who can access it and the data their employees will use with the tools. Lastly, is there a human touch — in the form of oversight of the tools' outputs? This addition can help account for accuracy and bias.
Generative AI tools understand text and instructions in context and can communicate them more humanly. They not only extract information but convert it into images and natural-sounding responses, making you feel like you're conversing with an informed coworker.
Fernando Schwartz, VP, data science and machine learning engineering, ADP
Glossary of key terms
Generative AI can be a helpful HR tool. Leaders should talk to their technical teams about implementing it and the opportunities it can create for their unique workplaces. They should arrive informed, as generative AI terminology can be challenging to understand. HR technology professionals on the vendor side may also use the terminology, so preparing for these discussions is essential. Key terms and phrases leaders might need to know include:
- Vector databases – a collection of vector representations (mathematical representations) of data objects, such as words, phrases and documents
- Embeddings – numerical representations of words or phrases that capture their semantic meanings
- Internal hosting – refers to running generative AI systems on servers owned and operated by the business using the systems
- External hosting – involves running generative AI systems on servers owned and operated by a third-party vendor
- Prompt engineering – refers to designing and refining queries, or prompts, for generative AI systems
- Fine-tuning – refers to adjusting a pretrained language model to better fit a specific task or domain
- Few-shot learning – refers to training language models to generate new text based on a small number of examples or prompts
- Context learning – refers to training a model to understand the context in which a query, or prompt, is made
- Pretraining – refers to training a language model on an extensive collection of text data before fine-tuning it on a specific task or dataset
By researching and understanding these terms, leaders can make more informed decisions about implementing generative AI.
Bottom line: Be an informed HR decision-maker
Both extractive and generative AI have their use cases. Ensure you understand their differences so you arrive informed at the decision-making table. Additionally, given the newness of generative AI, carefully consider how your HR vendor uses it in their products and services. Ask about their governance models, AI ethics policies, compliance strategies, data privacy and data security and what to consider when implementing generative AI technology.
"Partnering with an HR vendor that not only has a breadth of AI expertise but plenty of data and experience is crucial," Schwartz says. "It's important that you can trust where your AI solutions are being housed and how they're being managed."